healthSCAN utilizes near-infrared spectroscopy (NIRS) to measure three sites on the body; the inner forearm, the back of the hand and the fingernail. Coalescence and analysis of spectral data obtained from each site provides valuable information on the physical well-being of the user. A machine-learning algorithm combines these measurements, generating scientific, personalized and useful recommendations without a medical diagnosis.
Features used to Generate Recommendations
Glucose Status1: Glucose, or sugar, utilization is an essential component of the body’s energy storage and utilization system. Impaired glucose metabolism, may indicate a user would benefit from products containing more protein and fiber and fewer calories from sugar and other simple carbohydrates.
Body Fat: NIRS provides quick and non-invasive method for measuring body fat
2. Insight into body composition allows for the recommendation of products appropriate for the user’s body type, such as those designed for weight loss or weight gain.
Bone Quality: Bone quality is a highly dynamic system influenced by many factors evaluated by healthSCAN. Diminished bone quality is encountered frequently in the general population as well as in the fitness industry, particularly amongst endurance athletes. NIRS helps identify users that may benefit from supplements that support bone metabolism.
3,4
Tissue and Muscle Oxygenation: Analysis of tissue oxygenation provides insight into a user’s training status and recovery rate
5,6. Using this information, healthSCAN is able to recommend products that may enhance and optimize training and recovery.
Blood: Blood contains many features that are indicative of a user’s overall health, such as hemoglobin
9 and cholesterol
10. Using this data, healthSCAN may recommend products containing iron for a user with below average hemoglobin.
Skin: NIRS of the skin reveals several useful parameters such as skin thickness and hydration status
11. These measurements allow for beverage recommendations as well as moisturizers, cosmetics and oils.
healthSCAN and Tissue Spectroscopy
NIR spectroscopy has been used for decades in a variety of ways, from laboratory research to evaluation of samples such as food, oils, fabrics and other commodities. Until recently, expansion of NIR to the diverse needs of consumers has been limited by the cost and size of industrial spectrometers. healthSCAN uses the first hand-held NIR spectrometer to bring personalized health product recommendations to consumers.
At a molecular level, NIR spectroscopy emits a beam of photons into a sample. Different substances absorb and scanner NIR light to different degrees at various wavelengths. The spectrometer measures the proportion of light that is returned over a wide range of optical wavelengths, allowing the spectrometer to identify and measure the substances present within a sample. healthSCAN harnesses the molecular power of NIR spectroscopy through advanced machine learning algorithms, allowing detection of the presence of many physiologic substances that play a role in human health.
Recommendation without Diagnosis
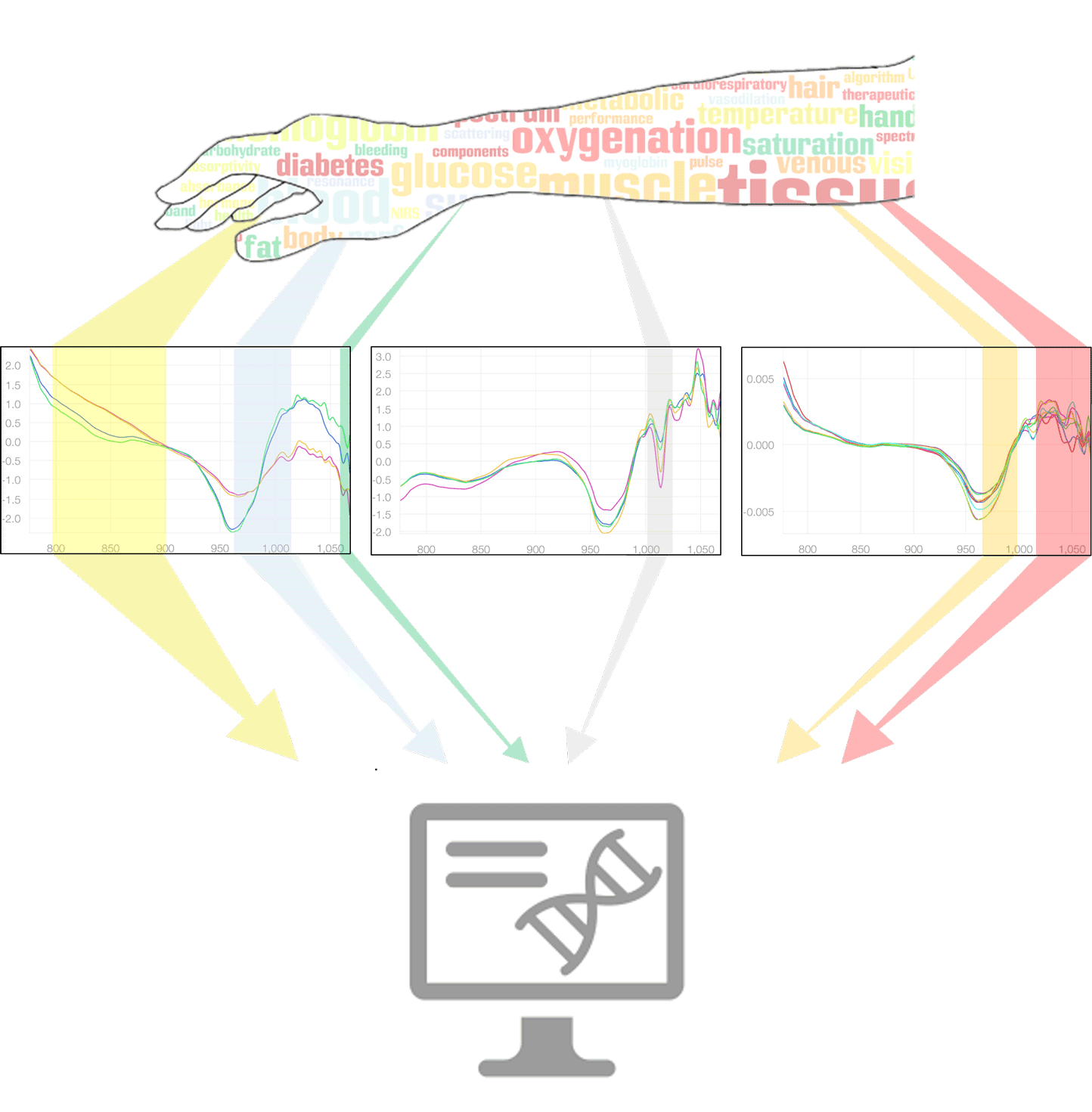
Comprehensive nutritional or health recommendations traditionally follow direct measurement of laboratory values, or quantities, such as blood glucose or cholesterol. Such measurement constitutes medical diagnosis, which requires physical examination by a doctor and/or regulated laboratory testing. Furthermore, direct, isolated measurement of these quantities by NIRS is often challenging. For example, the precise measurement of glucose is confounded by the presence of cholesterol, uric acid and glycated albumin, which absorb light in the same spectrum. In machine learning terminology, the 'latent quantities' combine to form the 'observed features' of spectrum.
healthSCAN can offer valuable recommendations without making a medical diagnosis because, unlike previous applications of NIRS, healthSCAN does not attempt to deconstruct the spectra into discrete underlying quantities. Rather, healthSCAN uses a novel, robust machine learning algorithm to analyze the 'observed features' while simultaneously recognizing the involvement of 'latent quantities'. The algorithm then uses this information to match consumers with the appropriate products for them.